MA介绍
由于微动脉瘤(MA)在糖尿病视网膜病变(DR)诊断中的作用,视网膜图像中的微动脉瘤检测目前是一个活跃的研究领域。
过去的大部分研究都集中在从视网膜图像中检测DR等级。其他研究集中在从视网膜图像中检测单个微动脉瘤。在我们的文献搜索中,我们发现已经提出了数十种微动脉瘤检测算法。为比较这些技术而对其进行评估成为一项具有挑战性的任务。这些论文中有很多是在私人数据集上评估他们的技术,这使得他们的结果难以验证。据我们所知,唯一公开的微动脉瘤检测数据集是由视网膜病变在线挑战赛发布的数据集(ROC数据集)[1]。ROC数据集包含100张图像,50张训练图像和50张测试图像。然而,这个数据集的基础真理只适用于训练图像。测试集的基本事实没有公布,因为它们是用来评估竞赛作品的性能的。此外,这个数据集的groundtruth已经引起了讨论[2-4],因为许多在图像中标记的MA候选人对观众来说是看不见的。这两个原因给那些最近开发的算法带来了困难,因为他们无法将他们的技术性能与那些已经报告了相同数据集性能的算法进行比较。由于这些原因,有必要建立一个可用于对微动脉瘤检测算法进行基准测试的数据集。
数据集介绍
The reasons mentioned above have motivated us to create our own groundtruth set that can be used to evaluate and compare the performance of multiple algorithms. A set of 20 images were chosen from this dataset to cover a wide range of retinopathy as shown in Table 1. The images were all either healthy or suffered from early-stage Diabetic Retinopathy and were good resolution images. There were no abnormalities such as laser scars in the images. The purpose of this selection was to have a dataset of ideal scenario images for assessment. This means that the results of the assessment on this dataset should produce the ideal performance of a given algorithm or technique. In other words, the dataset should identify the ‘peak performance’ of the algorithm being tested.
Retinopathy Grade | Number of Microaneurysms | Number of images (training) | Number of images (test) |
---|---|---|---|
DR0 | 0 | 8 | 8 |
DR1 | 1-5 | 3 | 4 |
DR2 | 6-14 | 3 | 3 |
DR3 | 15 | 2 | 1 |
TOTAL | 16 | 16 |
Table 1: The distribution of retinopathy in the dataset
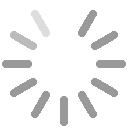
a) An image patch with MA candidates highlighted b) The image patch without the highlights c) The groundturth binary image patch generated
The images were groundtruthed by an expert grader. During the groundtruthing the grader marked all the microaneurysms that were visible to him. A circular marker was used rather than pixel-based marker [1]. Majority of the literature has relied on object-based metrics to measure the accuracy of detection. This is because it gives a more sensible measure of performance – indicating the amount of MA objects detected in the image relative to the total MA objects present. Furthermore, reliance on pixel-based metrics can be misleading due to the imbalance in proportion between very few MA pixels and a large number of background pixels.
The microaneurysm labeling was performed using imannotate, an open source MATLAB tool that we built to assist annotating images. We used the tool to label circles around each MA candidate that was labeled by the expert. In addition to marking each candidate, we also labeled each MA candidate using one of the following labels [1]: Obvious, Regular or Subtle based on their relative visibility and/or local contrast in the image. Close to Vessel is a label given to MA candidates that lie close to a blood vessel. According to [1] an MA candidate was marked as close to vessel if it lay around 1 MA diameter away from the vessel. We have stuck to this convention during our labeling process.
The images in the dataset belonged to the same resolution of 1440 x 960 px.
下载地址:https://github.com/motatoes/messidor-groundtruth/releases
数据引用:
Habib, M. M., et al. “Microaneurysm detection in retinal images using an ensemble classifier.” Image Processing Theory Tools and Applications (IPTA), 2016 6th International Conference on. IEEE, 2016.
本站原创,如若转载,请注明出处:https://www.ouq.net/2360.html